This report is based on 664 members of Pew Research Center’s American Trends Panel (ATP) who consented to share their username for research purposes, provided a verifiable TikTok handle and whose list of followed accounts is publicly visible on their profile. Refer to our earlier report methodology for more details about the ATP and our handle collection and validation process.
Identification and data collection from followed accounts
For each of the TikTok accounts belonging to a respondent in our study, we first collected the full list of the accounts they follow by going into their profile page, viewing the accounts listed on their following list, and then attempted to collect their account information.3 Once these individual account lists were consolidated to remove duplicates, we were left with a total of 227,946 distinct followed accounts.4
For each of the followed accounts, we used an automatic web scraping tool to go to the account’s page and collect the following information:
- Profile metadata, such as the number of followers it has and the number of videos they have posted
- Any information in the “bio” field of the account
- For accounts with 5,000 or more followers5 and that were publicly accessible (n=132,538), we also collected video, image and metadata information for the five most recent posts available on the account.6
This data collection took place in multiple stages. The initial followed account collection was collected from April 8 to 16, 2024, while the collection for their most recent videos happened between June 14 and June 20, 2024.7
Content analysis and categorization of the accounts with more than 5,000 followers followed by U.S. adult TikTok users were performed in two steps:
- First, we used a series of audio-to-text and video-to-text machine learning models to process and transcribe the content we had collected for each account. This information was all combined with profile details from the account to create a single text description that provides a snapshot of the account and its recent content.
- Next, we passed each account text description to OpenAI’s GPT-4o and GPT-4o-mini models along with an instruction prompt describing our codebook categories to obtain relevant classifications and labels for each account.
These steps are described in more detail below.
Consolidating profile and video details into account descriptions
For each account, we analyzed the five most recent posts available on the profile. In addition to metadata (such as the title and description) for each post, for video posts we collected full video files (with audio) and thumbnail images, and for slideshow posts we collected all images in the slideshow. This information was then processed and combined according to the following steps:
- Audio from video files was extracted and passed to an Audio Spectrogram Transformer model finetuned on the AudioSet dataset. This AST model inputs audio sequences, distinguishes speech from music, and then provides additional labels for the clip using a broad ontology of everyday sound types.
- For videos where the AST model identified “speech” as the primary audio label, the full audio from the video was then passed to OpenAI’s whisper transcription model. For a balance of accuracy and fast processing time, we used the 769M-parameter “medium” version of this model. On English-language speech, Whisper performs speech recognition and transcription. On speech in languages other than English, the model also performs translation and returns English-language transcriptions.
- All thumbnail images and slideshow images were passed through an optical character recognition (OCR) system using the python library EasyOCR. This OCR pass identified and extracted any text that could be read in the images.
- All thumbnail images and slideshow images were also passed through moondream2, a lightweight vision language model that can perform text generation conditioned on an image. We used this model to produce short descriptions of the subject of each image.
Once all posts collected for a given account were processed in this way, the resulting information was combined into a single account description text using the following template:
ACCOUNT URL: https://www.tiktok.com/@<USERNAME FROM PROFILE>
USERNAME: <USERNAME FROM PROFILE>
SCREEN NAME: <SCREEN NAME FROM PROFILE>
FOLLOWER COUNT: <FOLLOWER COUNT FROM PROFILE>
VIDEO COUNT: <VIDEO COUNT FROM PROFILE>
VERIFIED: <VERIFICATION STATUS FROM PROFILE>
BIO: <BIO FROM PROFILE>
RECENT HASHTAGS: <LIST OF HASHTAGS USED IN RECENT POSTS>
————————————————————————————–
This post is a video, captioned: “<POST TITLE/CAPTION>”
The thumbnail shows <DESCRIPTION OF IMAGE>
The following text can be read in the image: “<OCR OUTPUT>”
The audio used in the video can be classified as: <LIST OF AUDIO LABELS>
TRANSCRIPT: “<OUTPUT OF TRANSCRIPTION MODEL>”
————————————————————————————–
This post is a slide show, with <N IMAGES> images, captioned: “<POST TITLE/CAPTION>”
Here are descriptions of the images:
– <DESCRIPTION OF IMAGE 1>
– <DESCRIPTION OF IMAGE 2>
The following text can be read in the images: “<COMBINED OCR OUTPUTS>”
Classifying account descriptions
Once these account descriptions were produced for each account, we used the OpenAI batch API to generate relevant classifications and labels, using both the GPT-4o and GPT-4o-mini endpoints. These models were provided with each description in turn, along with a detailed instruction prompt explaining the codebook to be used for labeling and classification. (To see this prompt in full and the details of the codebook, refer to Appendix A).
The output of these models was validated using two hand-labeled validation sets. The first of these consists of the 194 accounts followed by the largest number of panelists. Accounts in this “popular accounts” sample were each triple-coded by a set of four expert annotators. The second validation set includes 200 randomly-sampled accounts, along with an additional oversample of 250 observations identified as predicted positive and negative cases for the “news” and “politics” categories, since these were particular categories of interest that occurred rarely in the data. Accounts in this “general accounts” sample were each coded between two and four times by the same set of four expert annotators. For both samples, ground truth labels were established from the set of multiple annotations per account using a Dawid-Skene aggregation model. (For full interrater reliability metrics, refer to Appendix B).
Considering all the available ground truth data together to calculate unified performance metrics for the models evaluated, we found that GPT-4o achieved an overall (micro averaged) accuracy score of 0.95, and an overall (micro averaged) F1 score of 0.77.
GPT-4o-mini’s performance was close to this level, with an overall (micro averaged) accuracy score of 0.94 and an overall (micro averaged) F1 score of 0.72. For each category, we used predictions from the model that performed the best at that specific task. When classifying accounts into non-mutually exclusive topical content categories, the model used predicted at least one of the same topical categories chosen by human annotators in 92% of cases. For organization subtypes, it overlapped with annotators in 79% of cases, and for public figure subtypes, it overlapped with annotators in 100% of cases. A detailed table of per-category metrics can be found in Appendix B.
Note that in cases where an individual user in the sample follows no other accounts, we consider the share of accounts in their following list that fall into each category to be zero as well.
Below are the full codebook categories that we considered for this study:
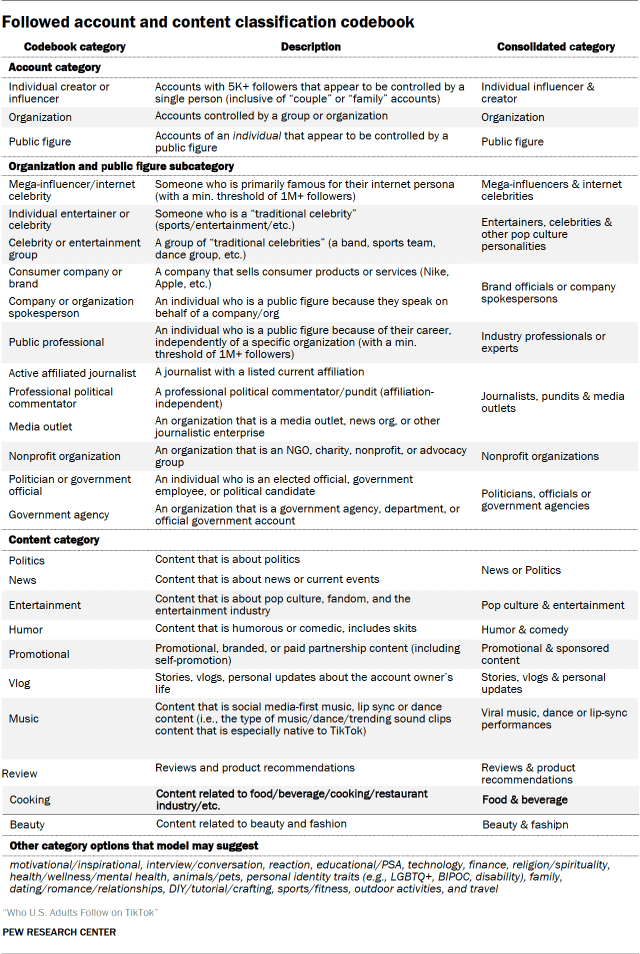