This study takes a close look at the guests who appeared on top-ranked podcasts in the United States in 2022. The methodology for how the 451 top-ranked podcasts and their content are identified, as well as how their episode data were collected, is available here. The vast majority of these podcasts (434 of 451) published at least one episode in 2022, with a total of 24,004 episodes. Roughly a third of these episodes (31%, or 7,423) included a guest, with a total of 7,212 guests appearing 10,996 times.
This is the latest report in Pew Research Center’s ongoing investigation of the state of news, information and journalism in the digital age, a research program funded by The Pew Charitable Trusts, with generous support from the John S. and James L. Knight Foundation.
Guest name extraction
All guests were identified through an analysis of published descriptions of podcast episodes. Those episode descriptions were collected from the Apple Podcasts and Spotify APIs in summer 2023.
There were 24,004 episodes collected from 434 podcasts included in the original study. The 17 podcasts that were not included either did not publish any episodes in 2022 or had removed episodes from those services when researchers collected episode descriptions.
Podcast episode descriptions are useful windows into the episode, as they often mention their guests by name. However, the language they use poses several challenges for an automated analysis.
First, podcast producers do not talk about their guests in consistent ways. For example, one description might introduce the guest with a phrase like “we are joined by [GUEST NAME]” while another might say “today [GUEST NAME] talks about….”
Second, even when the description mentions an individual by name, that person may not have been a guest. Instead, the podcast may have talked about this individual.
To overcome these difficulties, researchers used OpenAI’s Generative Pre-Trained Transformer model (GPT 3.5) to extract guest names from episode descriptions.
Using the three-part prompt structure below, we passed each episode description in turn to the gpt-3.5-turbo-0613 model endpoint:
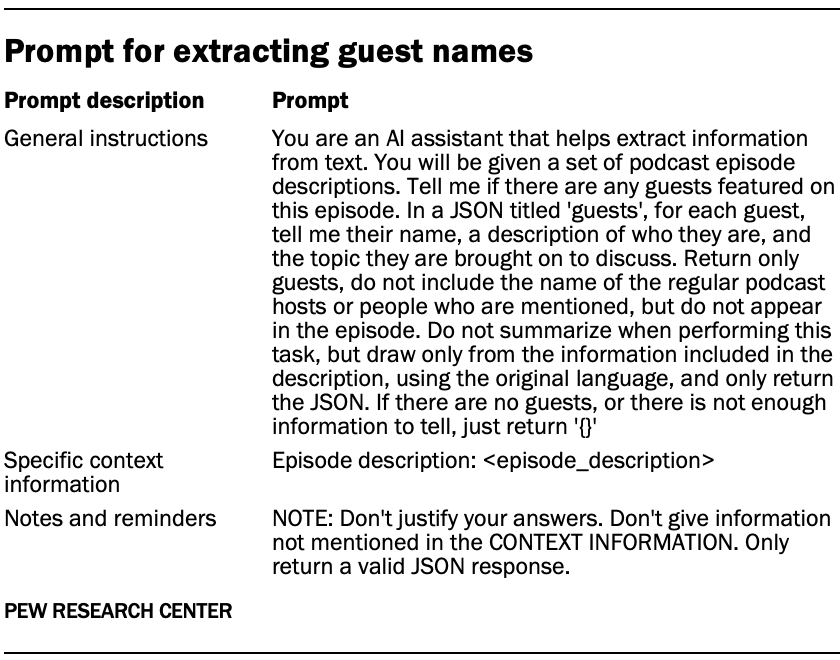
While GPT processed the vast majority of these episode descriptions without difficulty, the built-in content moderation guardrails in the GPT endpoint refused to give us any data for episode descriptions it determined were about sex or violence. After some testing, researchers used a different endpoint (the commercial OpenAI endpoint) that was less sensitive to those issues. Overall, 966 episodes, or about 4%, could not be evaluated because of the content moderation guardrails.
Validation of GPT guest name results
Researchers then validated the output from GPT using a pair of trained human coders, who validated the output independently. There were two measures evaluated: Has guests, or whether an episode description included a guest(s) or not; and Guest names, or the list of guests. In other words, researchers were verifying that GPT didn’t miss any guests and that the guests it identified were correct.
These human coders were trained to identify guests in episode descriptions. To confirm that coders were interpreting this task the same way, we measured intercoder agreement and Cohen’s kappa across both variables:
- Has guests: 97% agreement; 0.91 kappa
- Guest names: 96% agreement; 0.76 kappa
After training, the human coders evaluated a subset of 9% of the GPT output:
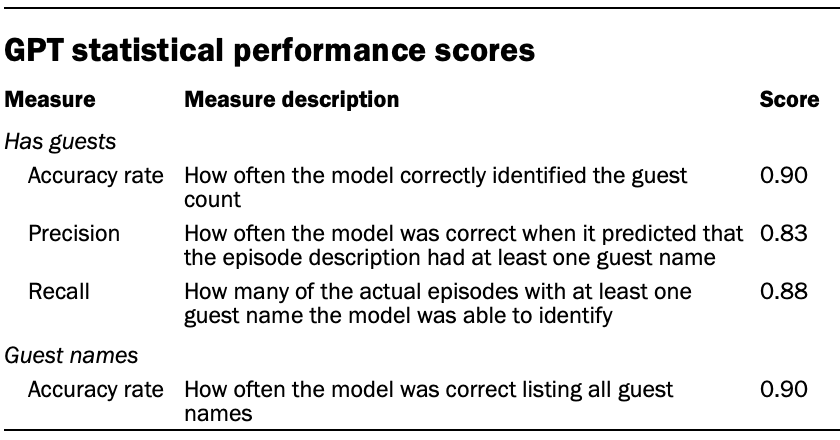
Data cleaning
As the metrics show, GPT did an acceptable job extracting the raw names of guest descriptions. But there were still several cleaning steps that needed to be performed:
- First, a small set (6%) of identified names only had a single name – which in many cases was not enough information to identify the guest.
- Second, the same identified name can refer to two different people – multiple people might be named John Smith, for instance.
- Finally, the same person may appear under different names – such as a person who goes by Elizabeth Smith and Lizzy Smith.
To identify any guests that needed this cleaning, researchers first removed any guests who were clearly a host or co-host on the podcast, and then hand-coded 966 guest names that fit those criteria. The final list included 7,212 guests.
Network analysis
To provide insight into the kinds of podcasts certain guests appeared on, researchers used network analysis methods that show guest sharing patterns across podcasts. About half (52%) of top-ranked podcasts (N=225) shared at least one guest with at least one other podcast.
These podcasts were analyzed using the walktrap community detection algorithm in R’s igraph package. To identify guest-sharing patterns for podcasts that commonly share guests, only podcasts that shared at least three guests with at least 12% overlap were included. This analysis uncovered five distinct groups (refer to the Appendix for a list of the podcasts in each group).
To illustrate the results of this network analysis, researchers used the network analysis tool Gephi to visualize a network map. In this map, each podcast is represented with a node, and the ties between podcasts indicate whether those podcasts share at least one guest. The thickness of the lines reflect the strength of these ties.