A study in which Facebook manipulated the news feeds to more than 600,000 users sent social media users into a cyber-swoon this week and spilled over into the mainstream media: “Facebook Tinkers With Users’ Emotions,” began the headline on the New York Times website.
But the controversy over what these researchers did may be overshadowing other important discussions, specifically conversations about what they really found—not much, actually—and the right and wrong way to think about and report findings based on statistical analyses of big data. (We’ll get to the ethics of their experiment in a moment.)
Because they are so large, studies based on supersized samples can produce results that are statistically significant but at the same time are substantively trivial. It’s simple math: The larger the sample size, the smaller any differences need to be to be statistically significant—that is, highly likely to be truly different from each other. (In this study, the differences examined were between those who saw more and those who saw fewer emotion-laden posts compared with a control group whose news feeds were not manipulated.)
And when you have an enormous random sample of 689,003, as these researchers did, even tiny differences pass standard tests of significance. (For perspective, a typical sample size in a nationally representative public opinion poll is 1,000.)
That’s why generations of statistics teachers caution their students that “statistically significant” doesn’t necessarily mean “really, really important.”
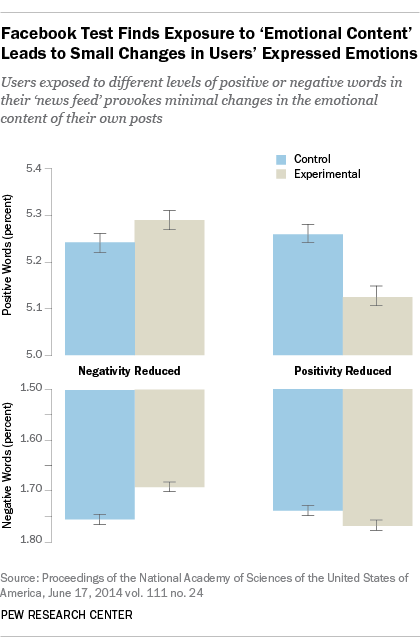
Consider the findings of the Facebook study in which they varied how many positive and negative posts from friends test subjects were allowed to see. Posts were determined to be positive or negative if they contained a single positive or negative word. Then, the test subject’s own use of positive and negative words in their status updates was monitored for a week. In all, test subjects posted a total 122 million words, four million of which were positive and 1.8 million negative.
As reported by the authors, the number of negative words used in status updates increased, on average, by 0.04% when their friends’ positive posts in news feeds were reduced. That means only about four more negative words for every 10,000 written by these study participants. At the same time, the number of positive words decreased by only 0.1%, or about one less word for every 1,000 words written. (As a point of reference, this post is a little more than 1,000 words long.)
Conversely, when negative posts were reduced, seven fewer negative words were used per 10,000, and the number of positive words rose by about six per 10,000.
Based on these results, the authors concluded in their published study that their “results indicate that emotions expressed by others on Facebook influence our own emotions, constituting experimental evidence for massive-scale contagion via social networks.”
But do these tiny shifts, even if they are real, constitute evidence of an alarming “massive-scale contagion”? Of course, importance is in the eye of the beholder. For some, these miniscule changes may be cause for alarm. But for others, they’re probably just meh.
One of the authors seems to have had second thoughts about the language they used to describe their work. In a Facebook post written in response to the controversy, Adam D. I. Kramer acknowledged, “My coauthors and I are very sorry for the way the paper described the research.”
He also suggested that, even with their huge sample, they did not find a particularly large effect. The results, he wrote, were based on the “minimal amount to statistically detect it — the result was that people produced an average of one fewer emotional word, per thousand words, over the following week.”
Critics have raised other questions, notably The Atlantic and Wired magazine, which questioned whether reading positive posts directly caused the Facebook user to use more positive words in their subsequent updates.
But is what Facebook did ethical? There is a good amount of discussion about whether Facebook was transparent enough with its users about this kind of experimentation. They did not directly inform those in the study that they were going to be used as human lab rats. In academic research, that’s called not obtaining “informed consent” and is almost always a huge no-no. (Facebook claims that everyone who joins Facebook agrees as part of its user agreement to be included in such studies.)
The question is now about how, sitting on troves of new social media and other digital data to mine for the same kind of behavioral analysis, the new rules will need to be written.
Experimental research is rife with examples of how study participants have been manipulated, tricked or outright lied to in the name of social science. And while many of these practices have been curbed or banned in academe, they continue to be used in commercial and other types of research.
Consider the case of the “Verifacitor,” the world’s newest and best lie detector—or at least that’s what some participants were told in this study conducted by researchers at the University of Chicago’s National Opinion Research Center in the mid-1990s.
The test subjects were divided into two groups. Members of the control group were asked to sit at a desk where an interviewer asked questions about exercise habits, smoking, drug use, sexual practices and excessive drinking.
The other test subjects answered the same questions while being hooked up by electrodes to the Verifacitor, described by the operator as a new type of lie detector. (In fact, it was just a collection of old computer components the researchers had lying around.)
To further enhance truth-telling, each participant was told before the formal interview began that the operator needed to calibrate the machine. So the participant was told to lie randomly in response to demographic questions about themselves that had been asked earlier on a screening questionnaire. (Questions like: Are you married? Did you finish high school? etc.).
Of course the interviewer had been slipped the correct answers so she immediately identified a bogus response, much to the amazement of the test subject.
Well you can guess what happened. Fully 44% of those in the Verifacitor group acknowledged they had ever used cocaine compared with 26% in the control group. Fully twice the proportion reported using amphetamines (39% vs. 19%), using other drugs (39% vs 19%) and drinking more alcohol than they should (34% vs. 16%).
In other words, social science research has a long history of manipulation. Will it learn from its past?