To analyze image search results for various occupations, researchers completed a four-step process. First, they created a list of U.S. occupations based on Bureau of Labor Statistics (BLS) data. Second, they translated these occupation search terms into different languages. Third, the team collected data for both the U.S. and international analysis from Google Image Search and manually verified whether or not the image results were relevant to the occupations being analyzed. Finally, researchers deployed a machine vision algorithm to detect faces within photographs, and then estimate whether those faces belong to men or women. The aggregated results of those predictions are the primary data source for this report.
Constructing the occupation list
Because researchers wanted to compare the gender breakdown in image results to real-world gender splits in occupations, the team’s primary goal was to match the terms used in Google Image searches with the titles in BLS as closely as possible.
But the technical language of the BLS occupations sometimes led to questionable search results. For example, searches for “eligibility interviewers, government programs” returned images from a small number of specialized websites that actually used that specific phrase, biasing results toward those websites’ images. So, the research team decided to filter out highly technical terms, using Google Trends to assess relative search popularity, relative to a reference occupation (“childcare worker”).
The query selection process for the U.S. analysis involved the following steps:
- Start with the list of BLS job titles in 2017.
- Exclude occupations that do not have information about the fraction of women employed. For example, “credit analysts” did not have information about the fraction of women in that occupation.
- Filter out occupations that do not have at least 100,000 workers in the U.S.
- Remove all occupations with ambiguous job functions (“all other,” “Misc.”).
- Split all titles with composite job functions into individual job titles (For example, “models and demonstrators” to “models,” “demonstrators”).
- Change plural words to singular (“models” to “model”) to standardize across occupations.7
- Manually inspect the list to ensure that the occupations were comprehensible and likely to describe human workers. This involved removing terms that might not apply to humans (such as tester, sorter) based on the researchers’ review of Google results.
- Use Google Trends to remove unpopular or highly technical job titles. Highly technical job titles like “eligibility interviewers, government programs” are searched for less frequently than less technical titles, such as “lawyer.” Accordingly, researchers decided to remove technical terms in a systematic fashion by comparing the relative search intensity of each potential job title against that of a reasonably common job title.8 The research team compared the search intensity results for each occupation with the search intensity of “childcare worker” using U.S. search interest in 2017. Any terms with search intensity below “childcare worker” were removed from the list of job titles. The reference occupation “childcare worker” was selected after researchers manually inspected the relative search popularity of various job titles and decided that “childcare worker” was popular enough that using it as a benchmark would remove many highly technical search terms.
The global part of the analysis uses a different list of job titles meant to capture more general descriptions of the same occupations. The steps to create that list include:
- Start with the list of BLS job titles that had at least 100,000 people working in the occupation in the U.S.
- Remove all occupations with ambiguous job functions (“all other”, “Misc.”).
- Split all titles with composite job functions into individual job titles (For example, “models and demonstrators” to “models,” “demonstrators”).
- Change plural words to singular (“models” to “model”) to standardize across occupations.
- Manually inspect the list to ensure that the occupations were comprehensible and likely to describe human workers. This involved removing terms that might not apply to humans (such as tester, sorter) based on manual review of Google results.
- Replace technical job titles with more general ones when possible to simplify translations and better represent searches. For example, instead of searching for “postsecondary teacher,” the team searched for “professor,” and instead of “chief executive,” the team used “CEO.”
- Use Google Trends to filter unpopular job titles relative to a reference occupation (“childcare worker”), following the same procedure described above. Any terms with search intensity below the search intensity of the reference occupation were removed.
- Select the top 100 terms with the most popular search intensity in Google in the U.S. within the past year.
- Translate each job title and determine which form to use when multiple translations were available.
Translations
To conduct the international analysis, the research team chose to examine image results within a subset of G20 countries, which collectively account for 63% of the global economy. These countries include Argentina, Australia, Brazil, Canada, France, Germany, India, Indonesia, Italy, Japan, Mexico, Russia, Saudi Arabia, South Africa, South Korea, Turkey, the United Kingdom and the United States. The analysis excludes the European Union because some of its member states are included separately and China because Google is blocked in the country. Researchers used the official language of each country for each search (or the most popular language if there were multiple official languages), and worked with a translation service, cApStAn, to develop the specific search queries.
To approximate search results for each country, researchers adjusted Google’s country and language settings. For example, to search jobs in India, job titles were queried in the Hindi language with the country set to India. Several countries in the study share the same official language; for example, Argentina and Mexico both have Spanish as their official language. In these cases, researchers executed separate queries for each language and country combination. The languages used in the searches were: Modern Standard Arabic, English, French, German, Hindi, Indonesian, Italian, Japanese, Korean, Portuguese, Russian, Spanish and Turkish.
Many languages spoken in these countries have gender-specific words for each occupation term. For example, in German, adding “in” to the end of the word “musiker” (musician) gives a female connotation to the word. However, the word “musiker” may not exclusively imply “male musician,” and it is not the case that only male musicians can be referred to as “musiker.” In consultation with the translation team, researchers identified the gender form of each job that would be used when a person of unknown gender is referenced, and searched for those terms. The male version is the default choice for most languages and occupations, but the translation team recommended using the feminine form for some cases when it was more commonly used. For example, researchers searched for “nurse” in Italian using the feminine term “infermière” rather than the masculine “infirmier” on the advice of the translation team.
In addition, some titles do not have a directly equivalent title in another language. For example, the job term “compliance officer” does not have an Italian equivalent. Finally, the same translated term can refer to different occupations in some languages. As a result, not all languages have exactly 20 search terms. Jobs lacking an equivalent translation in a given language were excluded.
Data collection
To create the master dataset used for both analyses, researchers built a data pipeline to streamline image collection, facial recognition and extraction, and facial classification tasks. To ensure that a large number of images could be processed in a timely manner, the team set up a database and analysis environment on the Amazon Web Service (AWS) cloud, which enabled the use of graphics processing units (GPUs) for faster image processing. Building this pipeline also allowed the researchers to collect additional labeled training images relatively quickly, which they leveraged to increase the diversity of the training set in advance of classifying the image search results.
Search results can be affected by the timing of the queries: Some photos could be more relevant during the time the query is executed, and therefore have a higher rank in the search results compared with searches at other times.
There are a number of filters users can apply to the images returned by Google. Under “Tools,” for example, users can signal to Google Image Search that they would like to receive images of different types, including “Face,” “Photo” and “Clip Art,” among other options. Users can also filter images by size and usage rights. For this study, researchers collected images using both the “photo” and “face” filter settings, but the results presented in this report use the “photo” filter only. Researchers made this decision because the “photo” filter appeared to provide more diverse kinds of images than the “face” filter, while also excluding clip art and animated representations of jobs.
Removing irrelevant queries
For occupations included across both the U.S. and international analysis term lists, some queries returned images that did not depict individuals engaged in the occupation being examined. Instead, they often returned images that showed clients or customers, rather than practitioners of the occupation, or depicted non-human objects. For example, the majority of image results for the term “physical therapist” showed individuals receiving care rather than individuals engaging in the duties associated with being a physical therapist.
To ensure the relevance of detected faces, researchers reviewed all of the collected images for each language, country and occupation combination. For the U.S. analysis, there were a total of 239 sets of images to review. For the international analysis, there were 1,800 sets of images to review. Queries were categorized into one of four categories based on the contents of the collected images.
- “Pass”: More than half of collected images depict only individuals employed in the queried occupation. Overall, 44% of jobs in the U.S. analysis and 43% of jobs in the global analysis fell into this category.
- “Fail”: The majority of collected images do not depict any face or depict faces irrelevant to the desired occupation. In many languages, the majority of collected images for the occupation “barber” depict only people who have been to a barber, rather than the actual barber. In the analysis of international search results, this includes queries that return images of an occupation different from that initially defined by the English translation. For example, the Arabic translation of “janitor” returns images of soccer goalies when queried in Saudi Arabia. Because the faces depicted in these images are not representative of the desired occupation, we categorize these queries as “fail.” A total of 31% of jobs in the U.S. analysis and 37% of jobs in the global analysis fell into this category.
- “Complicated”: The majority of collected images depict multiple people, some of whom are engaged in the queried occupation and some of whom are not. For example, the term “preschool teacher” and its translations often return images that feature not only a teacher but also students. These queries are categorized as “complicated” because of the difficulty in isolating the relevant faces. A total of 23% of jobs in the U.S. analysis and 17% of jobs in the global analysis fell into this category.
- “Ambiguous”: Some queries do not fall into the other categories, as there is no clear majority of image type or it is unclear whether the people depicted in the collected images are engaged in the occupation of interest. This may occur if the term has many definitions, such as “trainer,” which can refer to a person who trains athletes or various training equipment, or if the term has other usage in popular culture, such as the surname of a public figure (“baker”) or the name of a popular movie (“taxi driver”). Just 2% of jobs in the U.S. analysis and 3% of jobs in the global analysis fell into this category.
To minimize any error caused by irrelevance of detected faces in collected images, we remove all queries categorized as “fail,” “complicated” or “ambiguous” and only retain those queries categorized as “pass.”
Machine vision for gender classification
Researchers used a method called “transfer learning” to train a gender classifier, rather than using machine vision methods developed by an outside vendor. In some commercial and noncommercial alternative classifiers, “multitask” learning methods are used to simultaneously perform face detection, landmark localization, pose estimation, gender recognition and other face analysis tasks. The research team’s classifier achieved high accuracy for the gender classification task, while allowing the research team to monitor a variety of important performance metrics.
Face detection
Researchers used the face detector from the Python library dlib to identify all faces in the image. The program identifies four coordinates of the face: top, right, bottom and left (in pixels). This system achieves 99.4% accuracy on the popular Labeled Faces in the Wild dataset. The research team cropped the faces from the images and stored them as separate files.
Many images collected do not contain any individuals at all. For example, all images returned by Google for the German word “Barmixer” are images of a cocktail shaker product, even with the country search parameter set to Germany. To avoid drawing inference based on a small number of images, researchers included only queries that have at least 80 images downloaded and 50 images with at least one face detected in the analysis. Across different countries, the number of faces detected in the images varied. Hindi and Indonesian had the most detected faces. This means that their images tend to feature more people in them than other languages.
The table below summarizes the number of queries, number of images and number of faces detected. Overall, researchers were able to collect over 95% of the top 100 images that we sought to download.
Training the model
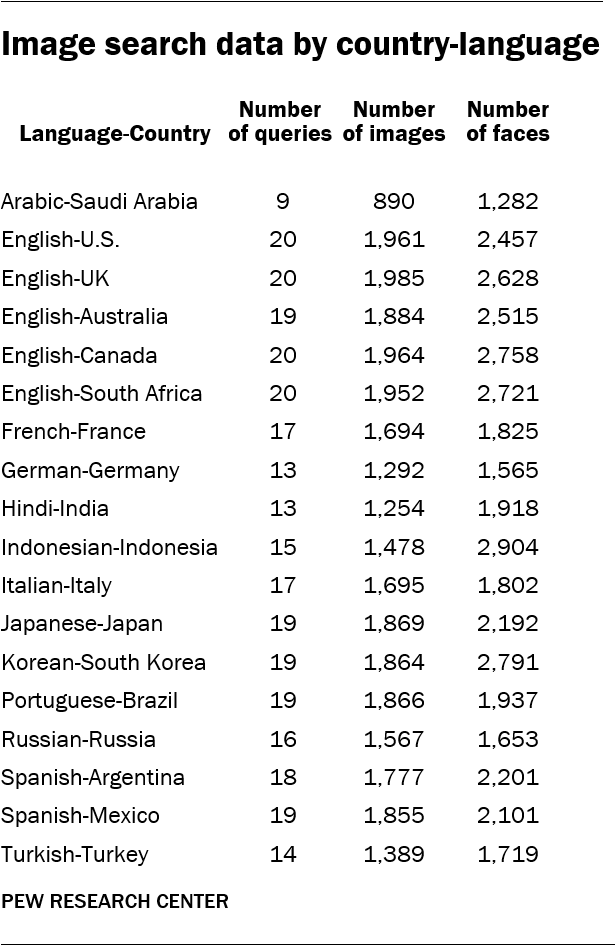
Recently, research has provided evidence of algorithmic bias in image classification systems from a variety of high-profile vendors.9 This problem is believed to stem from imbalanced training data that often overrepresents white men. For this analysis, researchers decided to train a new gender classification model using a more balanced image training set.
However, training an image classifier is a daunting task because collecting a large labeled dataset for training is very time and labor intensive, and often is too computationally intensive to actually execute. To avoid these challenges, the research team relied on a technique called “transfer learning,” which involves recycling large pretrained neural networks (a popular class of machine learning models) for more specific classification tasks. The key innovation of this technique is that lower layers of the pretrained neural networks often contain features that are useful across different image classification tasks. Researchers can reuse these pretrained lower layers and fine-tune the top layers for their specific application – in this case, the gender classification task.
The specific pretrained network researchers used is VGG16, implemented in the popular deep learning Python package Keras. The VGG network architecture was introduced by Karen Simonyan and Andrew Zisserman in their 2014 paper “Very Deep Convolutional Networks for Large Scale Image Recognition.” The model is trained using ImageNet, which has over 1.2 million images and 1,000 object categories. Other common pretrained models include ResNet and Inception. VGG16 contains 16 weight layers that include several convolution and fully connected layers. The VGG16 network has achieved a 90% top-5 accuracy in ImageNet classification.10
Researchers began with the classic architecture of the VGG16 neural network as a base, then added one fully connected layer, one dropout layer and one output layer. The team conducted two rounds of training – one for the layers added for the gender classification task (the custom model), and subsequently one for the upper layers of the VGG base model.
Researchers froze the VGG base weights so that they could not be updated during the first round of training, and restricted training during this phase to the custom layers. This choice reflects the fact that weights for the new layers are randomly initialized, so if we allowed the VGG weights to be updated it would destroy the information contained within them. After 20 epochs of training on just the custom model, the team then unfroze four top layers of the VGG base and began a second round of training. For the second round of training, researchers implemented an early-stopping function. Early stopping checks the progress of the model loss (or error rate) during training, and halts training when validation loss value ceases to improve. This serves as both a timesaver and keeps the model from overfitting to the training data.
In order to prevent the model from overfitting to the training images, researchers randomly augmented each image during the training process. These random augmentations included rotations, shifting of the center of the image, zooming in/out, and shearing the image. As such, the model never saw the same image twice during training.
Selecting training images
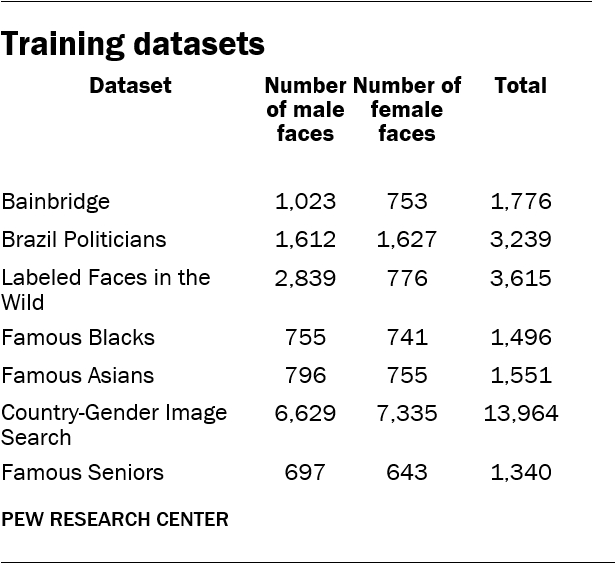
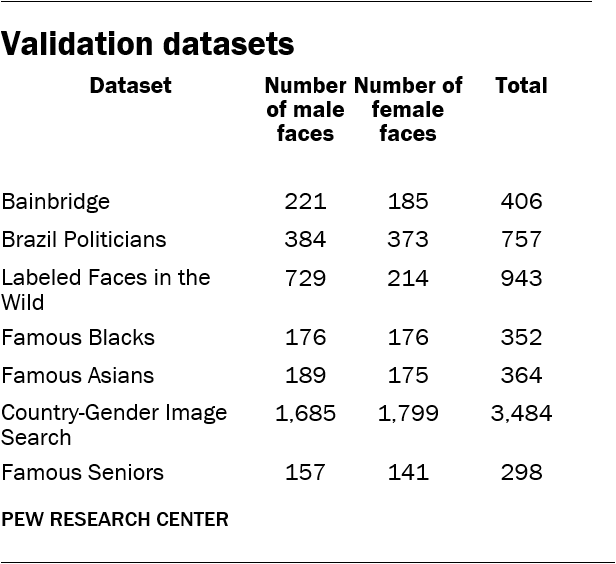
Image classification systems, even those that draw on pretrained models, require a substantial amount of training and validation data. These systems also demand diverse training samples if they are to be accurate across demographic groups. To ensure that the model was accurate when it came to classifying the gender of people from diverse backgrounds, researchers took a variety of steps. First, the team located existing datasets used by researchers for image analysis. These include the “Labeled Faces in the Wild” (LFW) and “Bainbridge 10K U.S. Adult Faces” datasets. Second, the team downloaded images of Brazilian politicians from a site that hosts municipal-level election results. Brazil is a racially diverse country, and that is reflected in the demographic diversity in its politicians. Third, researchers created original lists of celebrities who belong to different minority groups and collected 100 images for each individual. The list of minority celebrities focused on famous black and Asian individuals. The list of famous blacks includes 22 individuals: 11 men and 11 women. The list of famous Asians includes 30 individuals: 15 men and 15 women. Researchers then compiled a list of the most-populous 100 countries and downloaded up to 100 images of men and women for each nation-gender combination, respectively (for example, “French man”). This choice helped ensure that the training data included images that feature people from a diverse set of countries, balancing out the over-representativeness of white people in the training dataset. Finally, researchers supplemented this list with a set of 21 celebrity seniors (11 men and 10 women) to help improve model accuracy on older individuals. This allowed researchers to easily build up a demographically diverse dataset of faces with known gender and racial profiles.
Some images feature multiple people. To ensure that the images were directly relevant, a member of the research team reviewed each face manually and removed irrelevant or erroneous faces (e.g., men in images with women). Researchers also removed images that were too blurry, too small, and those where much of the face was obscured. In summary, the training data consist of 14,351 men and 12,630 women in images. The images belong to seven different datasets.
Model performance
To evaluate whether the model was accurate, researchers applied it to a subset equivalent to 20% of the image sources: a “held out” set which was not used for training purposes. The model achieved an overall accuracy of 95% on this set of validation data. The model was also accurate on particular subsets of the data, achieving 0.96 positive predictive value on the black celebrities subset, for example.
As a final validation exercise, researched used an online labor market to create a hand-coded random sample of 996 faces. This random subset of images overrepresented men – 665 of the images were classified as male. Each face was coded by three online workers. For the 924 faces that had consensus across the three coders, the overall accuracy of this sample is 88%. Using the value 1 for “male” and 0 for “female,” the precision and recall of the model were 0.93 and 0.90, respectively. This suggests that the model performs slightly worse for female faces, but that the rates of false positives and negatives was relatively low. Researchers found that many of the misclassified images were blurry, smaller in size, or obscured.
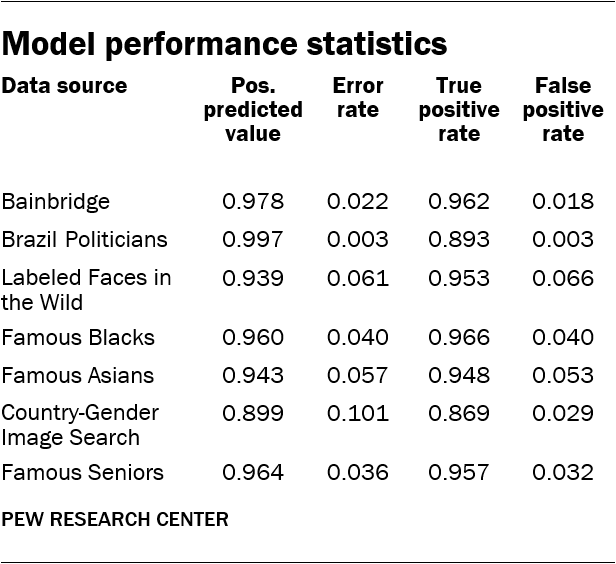
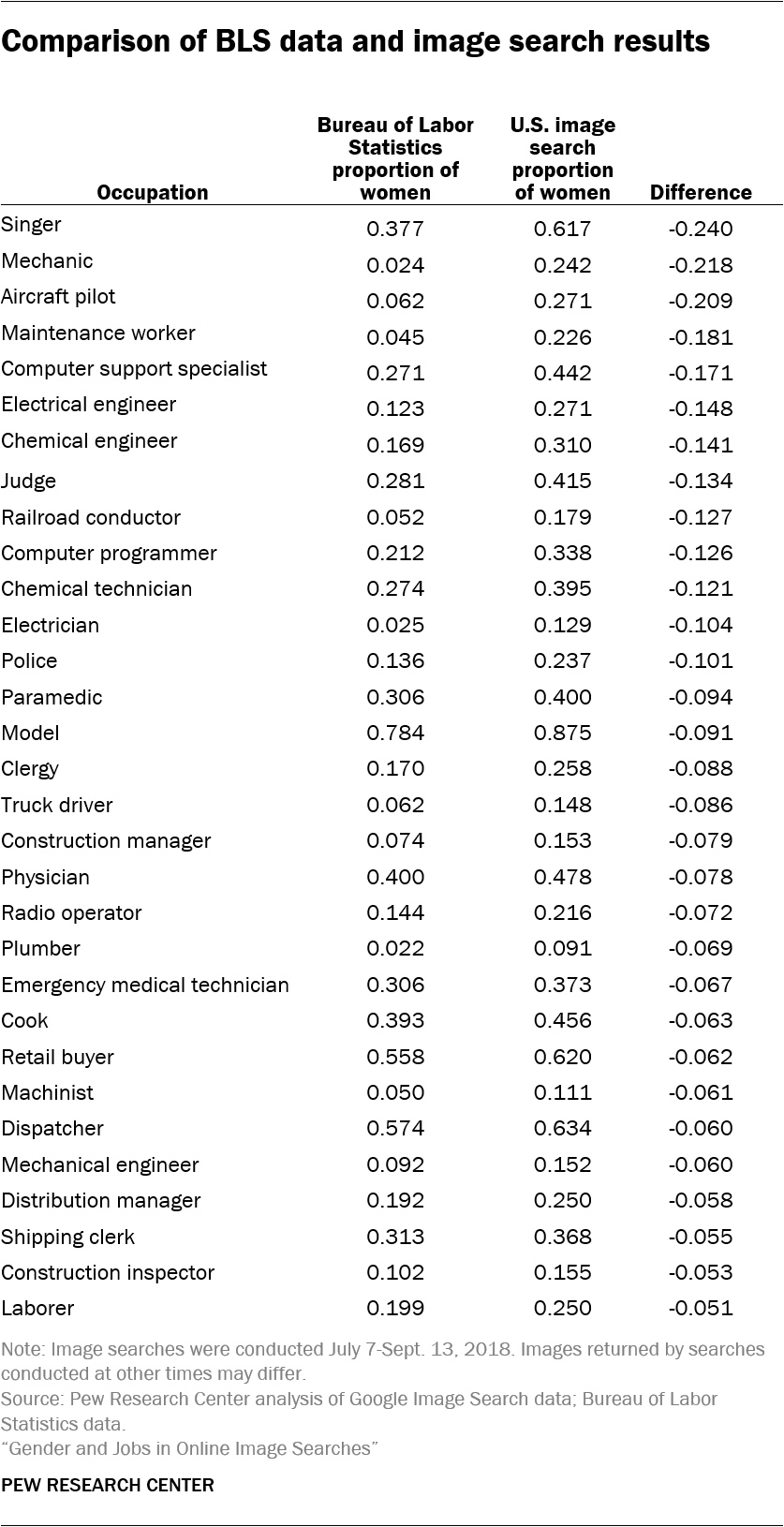
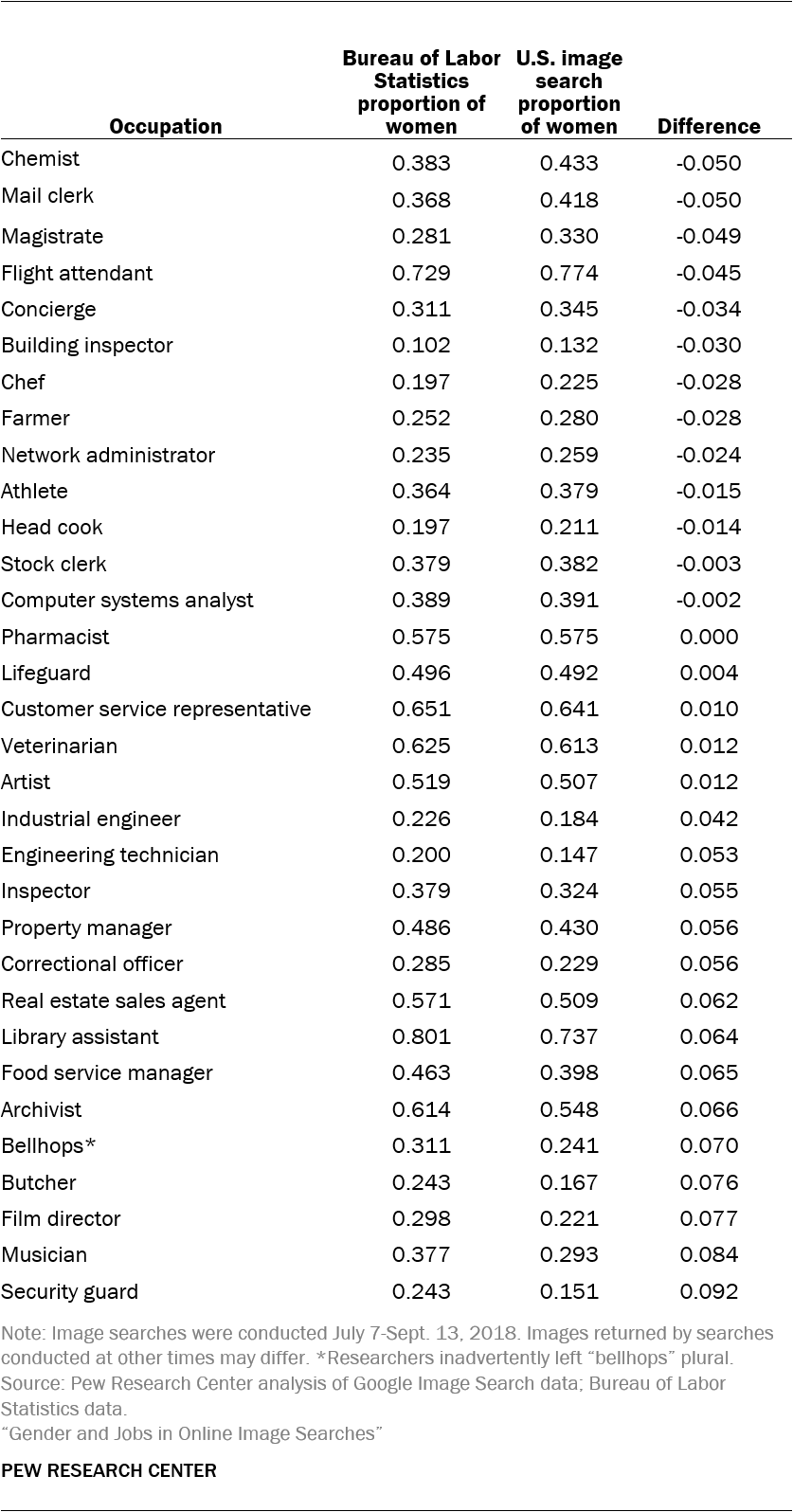
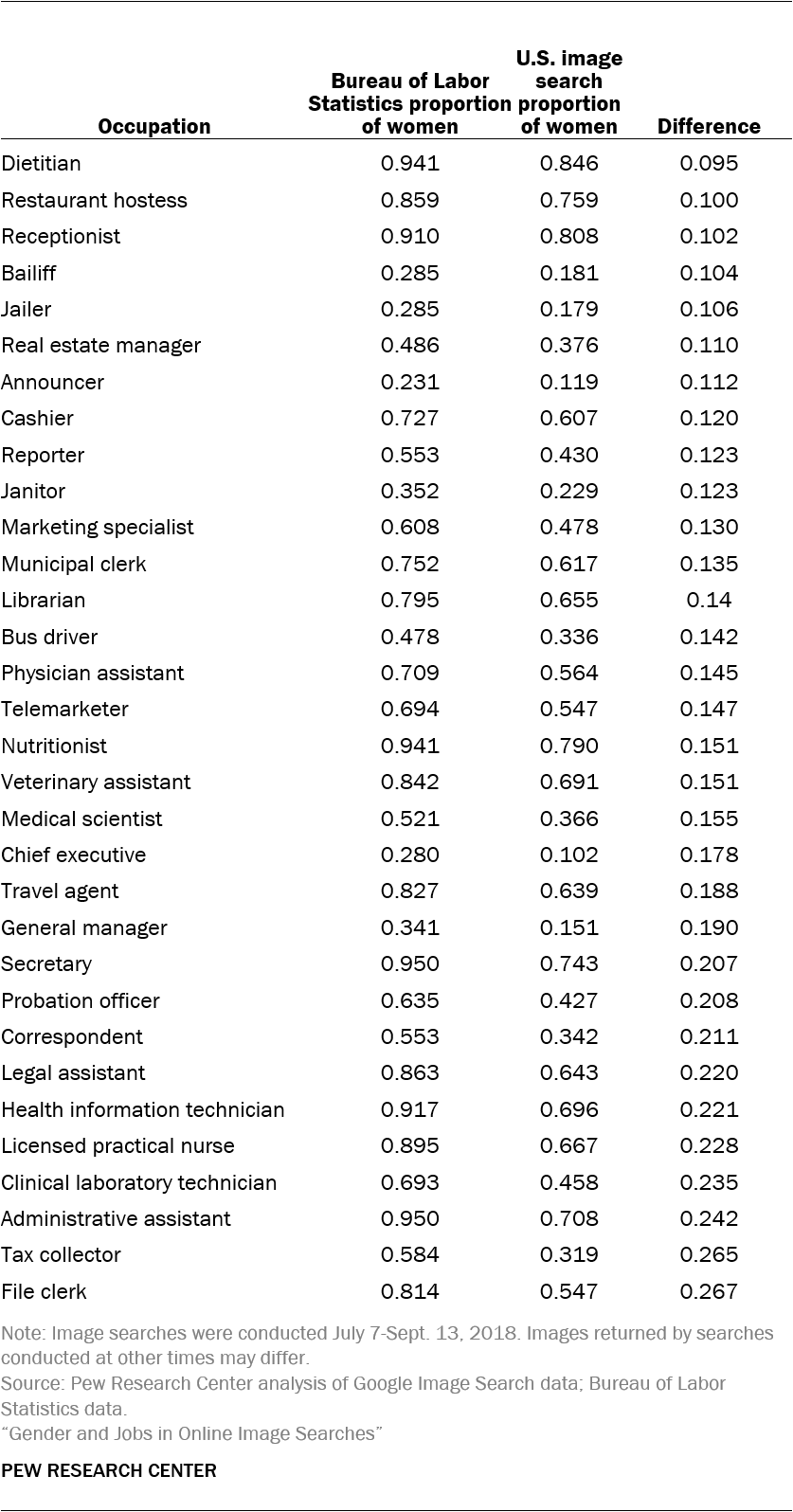
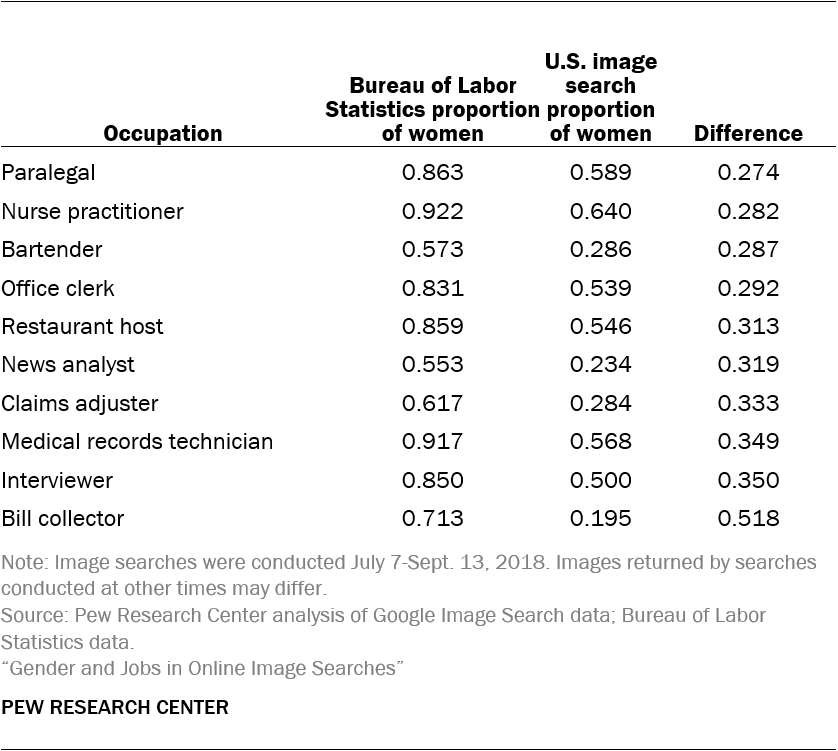
These results are also available in downloadable form here.